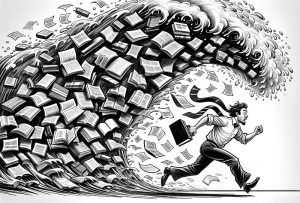
64 million scientific papers have been published since 1996 [1].
Assuming you can actually find the information you want in the first place—how can you organize your findings to be able to recall and use them later?
It’s not a trifling question. Discoveries often come from uniting different obscure pieces of information in a new way, possibly from very disparate sources.
Many software tools are used today for notetaking and organizing information, including simple text files and folders, Evernote, GitHub, wikis, Miro, mymind, Synthical and Notion—to name a diverse few.
AI tools can help, though they can’t always recall correctly and get it right, and their ability to find connections between ideas is elementary. But they are getting better [2,3].
One perspective was presented by Jared O’Neal of Argonne National Laboratory, from the standpoint of laboratory notebooks used by teams of experimental scientists [4]. His experience was that as problems become more complex and larger, researchers must invent new tools and processes to cope with the complexity—thus “reinventing the lab notebook.”
While acknowledging the value of paper notebooks, he found electronic methods essential because of distributed teammates. In his view many streams of notes are probably necessary, using tools such as GitLab and Jupyter notebooks. Crucial is the actual discipline and methodology of notetaking, for example a hierarchical organization of notes (separating high-level overview and low-level details) that are carefully written to be understandable to others.
A totally different case is the research methodology of 19th century scientist Michael Faraday. He is not to be taken lightly, being called by some “the best experimentalist in the history of science” (and so, perhaps, even compared to today) [5].
A fascinating paper [6] documents Faraday’s development of “a highly structured set of retrieval strategies as dynamic aids during his scientific research.” He recorded a staggering 30,000 experiments over his lifetime. He used 12 different kinds of record-keeping media, including lab notebooks proper, idea books, loose slips, retrieval sheets and work sheets. Often he would combine ideas from different slips of paper to organize his discoveries. Notably, his process to some degree varied over his lifetime.
Certain motifs emerge from these examples: the value of well-organized notes as memory aids; the need to thoughtfully innovate one’s notetaking methods to find what works best; the freedom to use multiple media, not restricted to a single notetaking tool or format.
Do you have a favorite method for organizing your research? If so, please share in the comments below.
References
[1] How Many Journal Articles Have Been Published? https://publishingstate.com/how-many-journal-articles-have-been-published/2023/
[2] “Multimodal prompting with a 44-minute movie | Gemini 1.5 Pro Demo,” https://www.youtube.com/watch?v=wa0MT8OwHuk
[3] Geoffrey Hinton, “CBMM10 Panel: Research on Intelligence in the Age of AI,” https://www.youtube.com/watch?v=Gg-w_n9NJIE&t=4706s
[4] Jared O’Neal, “Lab Notebooks For Computational Mathematics, Sciences, Engineering: One Ex-experimentalist’s Perspective,” Dec. 14, 2022, https://www.exascaleproject.org/event/labnotebooks/
[5] “Michael Faraday,” https://dlab.epfl.ch/wikispeedia/wpcd/wp/m/Michael_Faraday.htm
[6] Tweney, R.D. and Ayala, C.D., 2015. Memory and the construction of scientific meaning: Michael Faraday’s use of notebooks and records. Memory Studies, 8(4), pp.422-439. https://www.researchgate.net/profile/Ryan-Tweney/publication/279216243_Memory_and_the_construction_of_scientific_meaning_Michael_Faraday’s_use_of_notebooks_and_records/links/5783aac708ae3f355b4a1ca5/Memory-and-the-construction-of-scientific-meaning-Michael-Faradays-use-of-notebooks-and-records.pdf