I went down a rabbit hole this week using two symbols in LaTeX. The first was the Russian letter Sha (Ш, U+0248), and the second was the currency symbol for Bitcoin (₿, U+20BF).
Sha
I thought there would be a LaTeX package that would include Ш as a symbol rather than as a Russian letter, just as \pi
produces π as a symbol rather than as a Greek letter per se, but apparently there isn’t. I was surprised, since Ш is used in math for at least three different things [1].
When I post on @TeXtip how to produce various symbols in LaTeX, I often get a reply telling me I should simply paste in the Unicode character and use XeTeX. That’s what I ended up doing, except one does not simply use XeTeX.
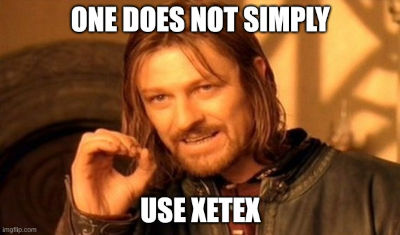
You have to set the font to one that contains a glyph for the character you want, and you have to use a font encoding package. I ended up adding these two lines to my file header:
\usepackage[T2A]{fontenc}
\usepackage{eulervm}
That worked, but only when I compiled with pdflatex
, not xelatex
.
Bitcoin
I ended up using a different but analogous tactic for the Bitcoin symbol. I used fontspec
, Liberation Sans, and xelatex
rather than fontenc
, Euler, and pdflatex
. These were the lines I added to the header:
\usepackage{fontspec}
\setmainfont{Liberation Sans}
Without these two lines I get the error message
Missing character: There is no ₿ (U+20BF) in font ...
I didn’t need to use ₿ and Ш in the same document, but the approach in this section works for both. The approach in the previous section will not work for ₿ because the Euler font does not contain a gylph for ₿.
Related posts
[1] The three mathematical uses of Ш that I’m aware of are the shuffle product, the Dirac comb distribution, and Tate–Shafarevich group.